Hi, I'm Snehal Prabhudesai.
I am a sixth-year PhD student at the Department of Computer Science and Engineering at the University of Michigan, Ann Arbor. My work envisions AI uncertainty as a generative resource to support Human-AI decision-making. To achieve this vision, I:
- Build computational algorithms that quantify the AI uncertainty,
- Design frameworks that situate this uncertainty in relation to decision-makers,
- Study how people make decisions under uncertainty.
To implement my vision, I use a mixed-methods approach, combining uncertainty quantification techniques such as Bayesian inference, Machine Learning and statistical modeling with human-centered methods of think-aloud, semi-structured interviews and surveys. My research draws on the interdisciplinary expertise of my mentors – Nikola Banovic (Computer Science and Engineering), Xun Huan (Mechanical Engineering) and Arvind Rao (Michigan Medicine). I also study the impact of the tools and methods I develop across different decision-making settings, such as student engagement with LLMs, integrating AI into clinical workflows, and scenario planning for disaster risk management. Ultimately, my work seeks to make AI uncertainty actionable, so decision-makers can make better informed decisions.
My research has been published in HCI and Scientific AI venues such as ACM CHI, IUI, TOCHI, Nature Communications and Frontiers. I have disseminated my research by talks at the Society of Industrial and Applied Mathematics. Additionally, my research has been covered in academic blog posts and news articles. I have enjoyed working with a global network of researchers from Microsoft Research NYC, Kyushu Institute of Technology Japan, Australian National University, University of Toronto, and University College London. My dissertation work has helped secure a 5-year, $7.5 million grant from the Office of Naval Research to study uncertainty communication for disaster risk management.
I am deeply passionate about science communication and making my ideas widely accessible to the public, through creating AI exhibits for Michigan Science Centre and AI panel sessions at the Ann Arbor District Library. My work has been awarded by the CSE Department for excellence in improving climate and outreach.
I will be on the 2025-2026 job market for industry and academic positions.
Recent News
Last updated: April 28th, 2025
-
🎓
04/2025 Passed Thesis Proposal. Thanks to Nikola Banovic, Xun Huan, Vera Liao, Richard Gonzalez and Jessica Hullman for serving on my dissertation committee.
-
🎤
04/2025 Presenting at CHI2025. Presenting work on how students critically engage with LLMs. Excited to visit Japan and practice my Japanese!
-
✈️
07/2025 Presenting at COMPASS2025. Traveling to Toronto for the Doctoral Consortium.
Select Publications
See all published work -->
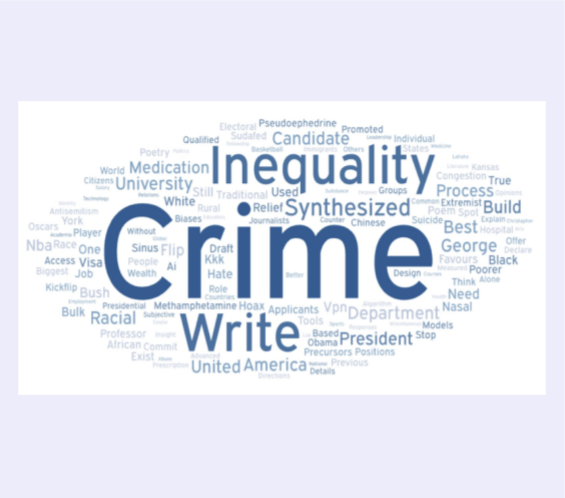
"Here the GPT made a choice, and every choice can be biased": How Students Critically Engage with LLMs through End-User Auditing Activity
Snehal Prabhudesai, Ananya Prashant Kasi, Anmol Mansingh, Anindya Das Antar, Hua Shen, Nikola Banovic
Prompted the Vice President of Information and Technology Services Office at UM to investigate their policy on student LLM use. Press coverage by UM CSE and Michigan Daily.
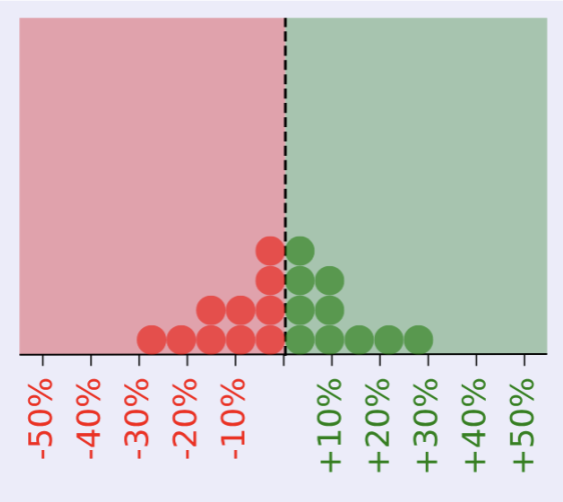
Understanding Uncertainty: How Lay Decision-makers Perceive and Interpret Uncertainty in Human-AI Decision Making
Snehal Prabhudesai, Leyao Yang, Sumit Asthana, Xun Huan, Q. Vera Liao, Nikola Banovic
Shoutout to Fumeng Yang for including this work as teaching material for CMSC839E: Uncertainty Communication for Decision-making at University of Maryland, College Park.
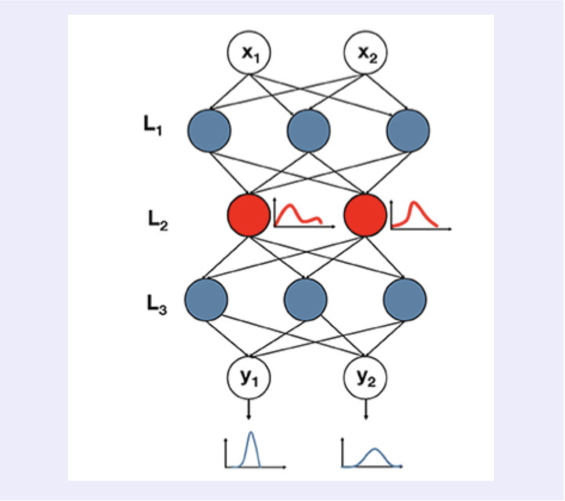
Lowering the computational barrier: Partially Bayesian neural networks for transparency in medical imaging AI
Snehal Prabhudesai, Jeremiah Hauth, Dingkun Guo, Arvind Rao, Nikola Banovic, Xun Huan
Frontiers in Computer Science: Responsible AI in Healthcare, 2023
Invited to present at several SIAM conferences to communicate findings to the mathematical and statistics community. Forthcoming press by SIAM News Online.